Artificial Intelligence (AI) is reshaping industries, from healthcare to finance, but its complex terminology often feels like a foreign language. If you’ve ever stumbled over terms like “neural networks,” “machine learning,” or “natural language processing,” you’re not alone. This guide simplifies AI jargon, empowering you to understand and engage with this transformative technology confidently.
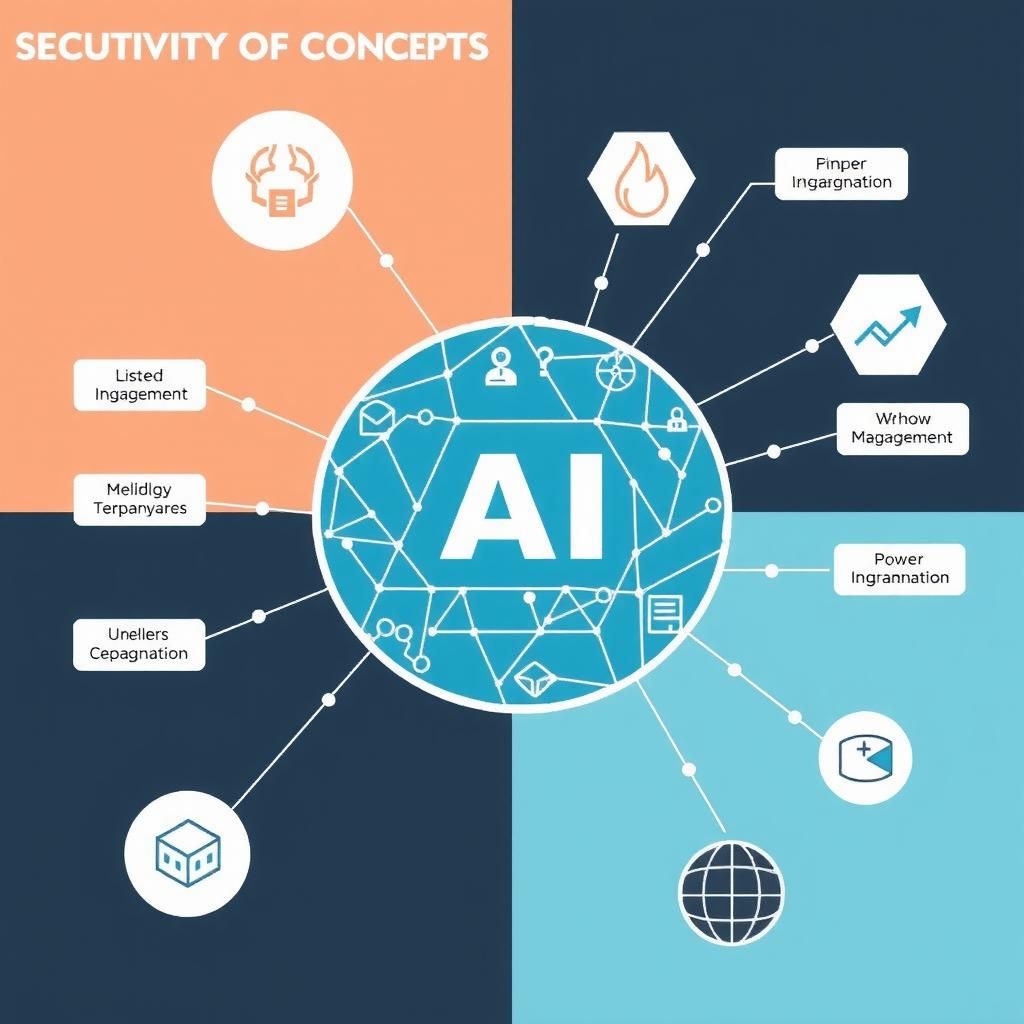
Table of Contents
1. Why Decoding AI Jargon Matters
AI is no longer confined to tech labs—it’s in smartphones, customer service chatbots, and even your Netflix recommendations. However, the language surrounding AI can alienate non-experts, creating barriers to adoption and understanding. By demystifying terms like “deep learning” or “algorithmic bias,” you’ll:
- Make informed decisions about AI tools.
- Engage in meaningful conversations about ethics and innovation.
- Navigate career opportunities in tech-driven fields.
2. Common AI Terms Explained (A-Z Glossary)
Let’s decode 20+ essential AI terms with simple definitions and relatable examples:
Algorithm
Definition: A step-by-step procedure for solving a problem or completing a task.
Example: A recipe is an algorithm for cooking a dish.
Machine Learning (ML)
Definition: A subset of AI where systems learn from data without explicit programming.
Example: Spotify’s “Discover Weekly” uses ML to recommend songs based on your listening history.
Neural Network
Definition: A system inspired by the human brain, designed to recognize patterns.
Analogy: Think of neurons as tiny decision-makers that collaborate to identify a cat in a photo.
Natural Language Processing (NLP)
Definition: Teaching machines to understand and respond to human language.
Example: Siri or Alexa answering your questions.
3. Breaking Down Complex AI Concepts with Analogies
What’s the Difference Between AI, ML, and Deep Learning?
AI: The umbrella term (e.g., “vehicles”).
ML: A subset of AI (e.g., “electric cars”).
Deep Learning: A specialized ML technique (e.g., “Tesla’s self-driving tech”).
How Do Neural Networks Work?
Imagine a team of detectives (neurons) analyzing clues (data) to solve a mystery (pattern recognition). Each detective passes insights to the next until they crack the case.
4. Real-World Examples of AI in Action
Healthcare: Diagnosing Diseases
IBM Watson analyzes medical data to suggest cancer treatments.
Jargon Decoded: “Predictive analytics” = forecasting health outcomes.
Retail: Personalized Shopping
Amazon’s recommendation engine uses ML to suggest products.
Jargon Decoded: “Collaborative filtering” = comparing your habits to similar users.
5. FAQs About AI Terminology
Q1: Is AI the same as robotics?
A: No. AI refers to software intelligence, while robotics involves physical machines. However, AI can power robots (e.g., self-driving cars).
Q2: What is “algorithmic bias”?
A: When AI systems reflect human prejudices in their training data. For example, a hiring tool favoring male candidates if trained on biased historical data.
Q3: Can AI replace human jobs?
A: AI automates tasks, not roles. It enhances productivity (e.g., doctors using AI for diagnostics) but requires human oversight.
6. Conclusion: Mastering AI Vocabulary
Understanding AI jargon isn’t about becoming an expert—it’s about breaking down barriers. With this guide, you’re equipped to:
- Ask better questions about AI tools.
- Advocate for ethical AI practices.
- Stay curious in a rapidly evolving field.
Pro Tip: Bookmark this guide or share it with peers struggling with terms like “GPT-4” or “computer vision”!