The Hidden Environmental Cost of Training LLMs: What's Being Done?
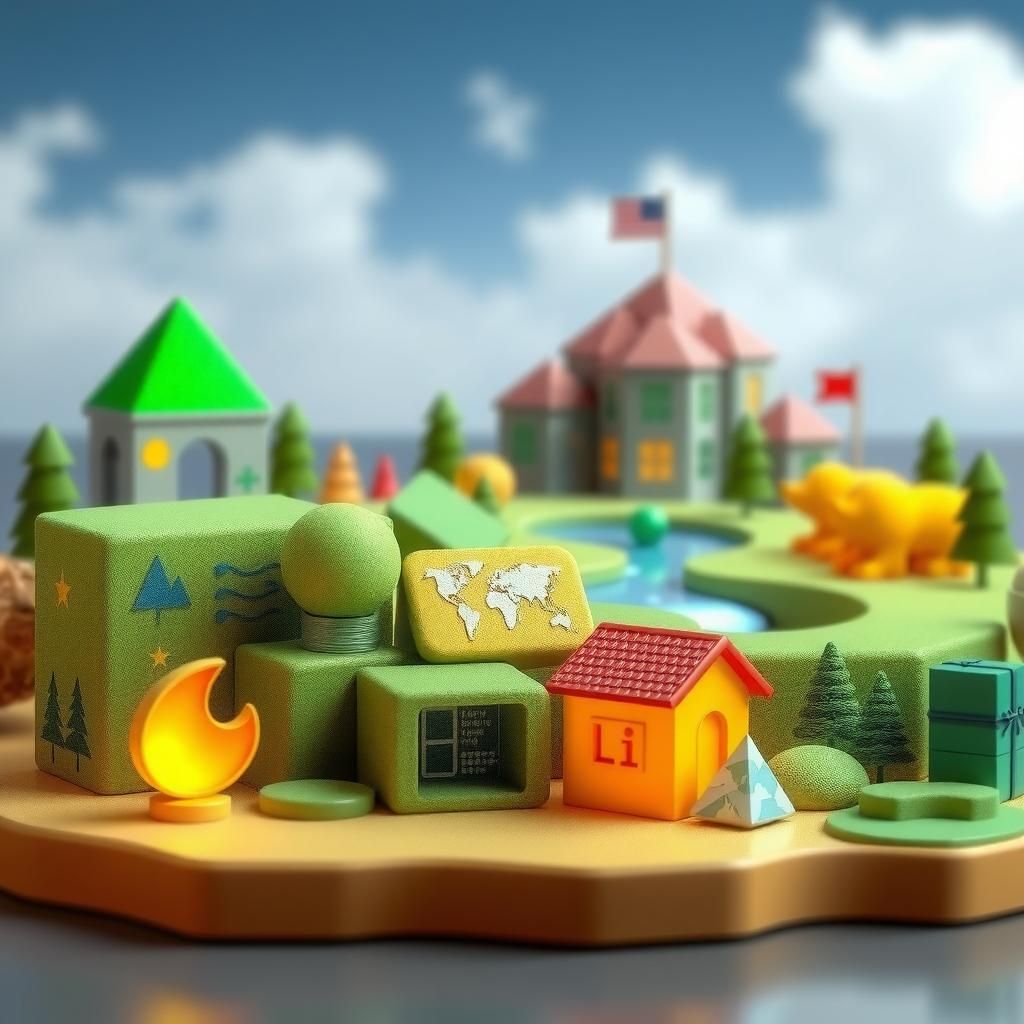
Introduction: The Dark Side of Digital Intelligence
In the dazzling world of artificial intelligence, Large Language Models (LLMs) have emerged as technological marvels, capable of understanding, generating, and manipulating human language with unprecedented sophistication. However, beneath the surface of these miraculous systems lies a stark and often overlooked reality: the substantial environmental toll of their development and training.
Researchers at Ethical Circuits have been at the forefront of investigating the ecological footprint of artificial intelligence, revealing a complex and challenging landscape of environmental impact.
Understanding the Carbon Footprint of LLMs
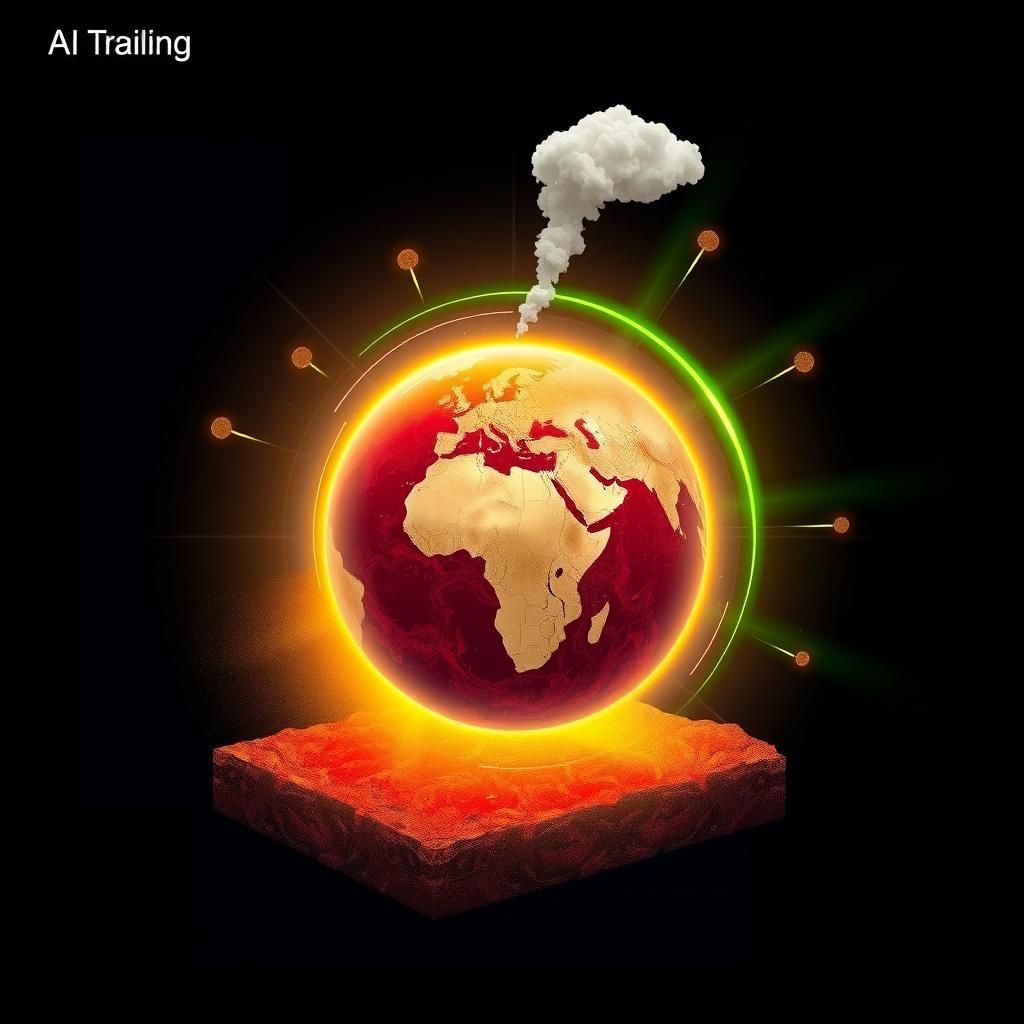
The Staggering Energy Consumption
Training a single large language model can consume an equivalent amount of energy to:
- 5-10 average American homes' annual electricity consumption
- Driving a passenger car more than 500,000 miles
- Producing over 300 tons of carbon dioxide emissions
According to a groundbreaking study by Nature , the computational resources required for training advanced AI models have been growing exponentially, presenting a significant environmental challenge.
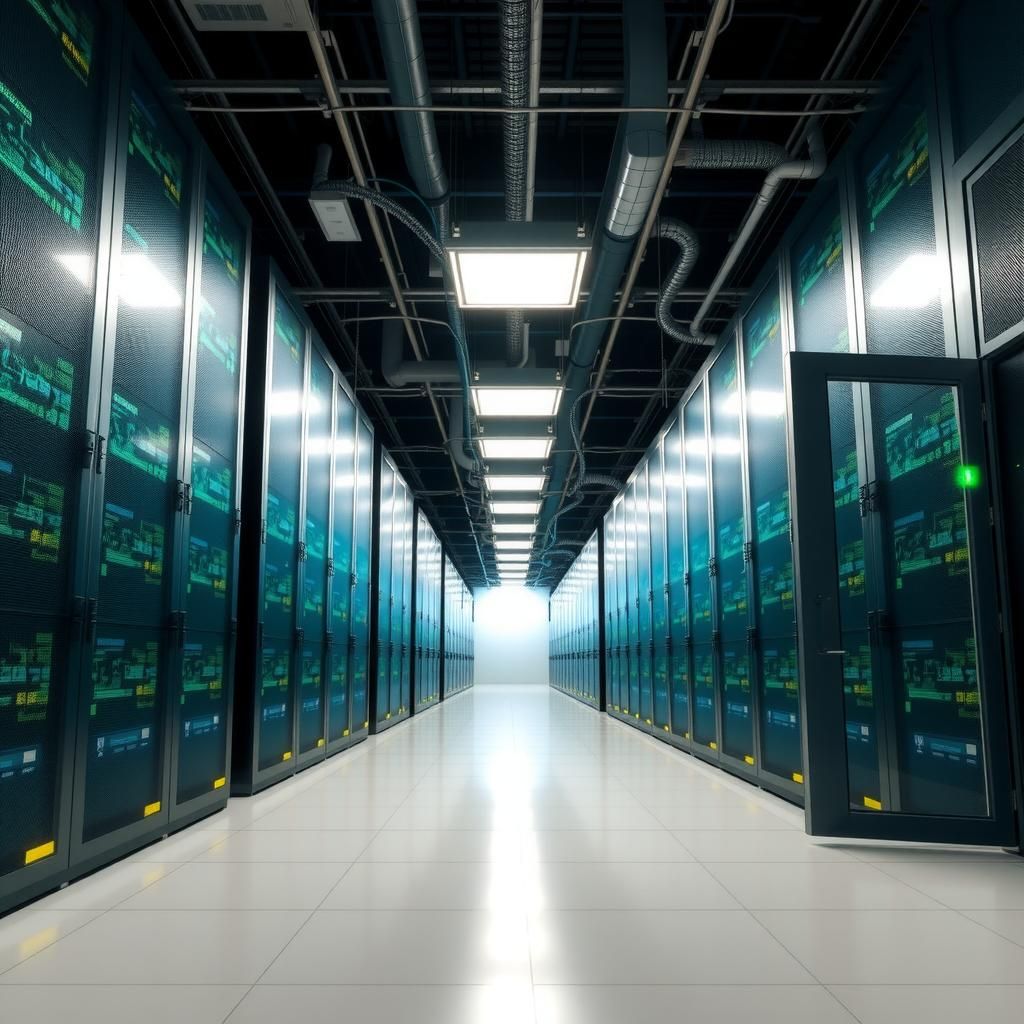
Breaking Down the Energy Consumption Process
The environmental cost of training LLMs can be attributed to several critical factors:
- Computational Intensity: Massive data centers running complex algorithms
- Hardware Requirements: High-performance GPUs and specialized computing infrastructure
- Cooling Systems: Extensive energy needed to prevent hardware overheating
- Data Transfer: Significant energy consumption in moving and processing vast datasets
The Global Context of AI's Environmental Impact

Research from MIT Technology Review suggests that the AI industry's carbon footprint could rival that of entire industrial sectors if left unchecked.
Comparative Environmental Analysis
LLM training emissions compared to other industries:
- Equivalent to annual emissions of small countries
- Potentially matching the carbon output of mid-sized manufacturing sectors
- Consuming more energy than some entire national electricity grids
Innovative Solutions and Mitigation Strategies
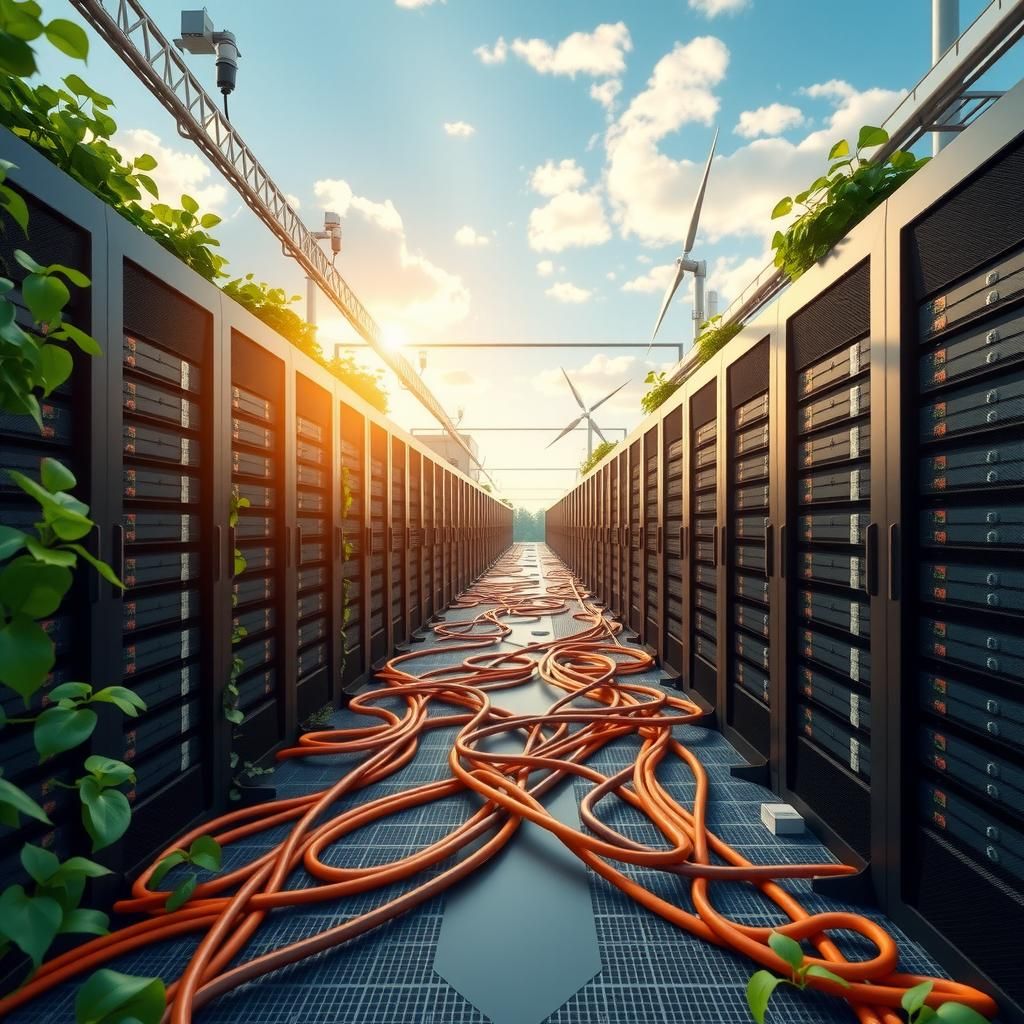
Green Computing Approaches
Leading technology companies and research institutions are developing multi-pronged strategies to address these environmental challenges:
- Renewable Energy-Powered Data Centers
- Energy-Efficient Hardware Design
- Advanced Cooling Technologies
- Carbon Offset Programs
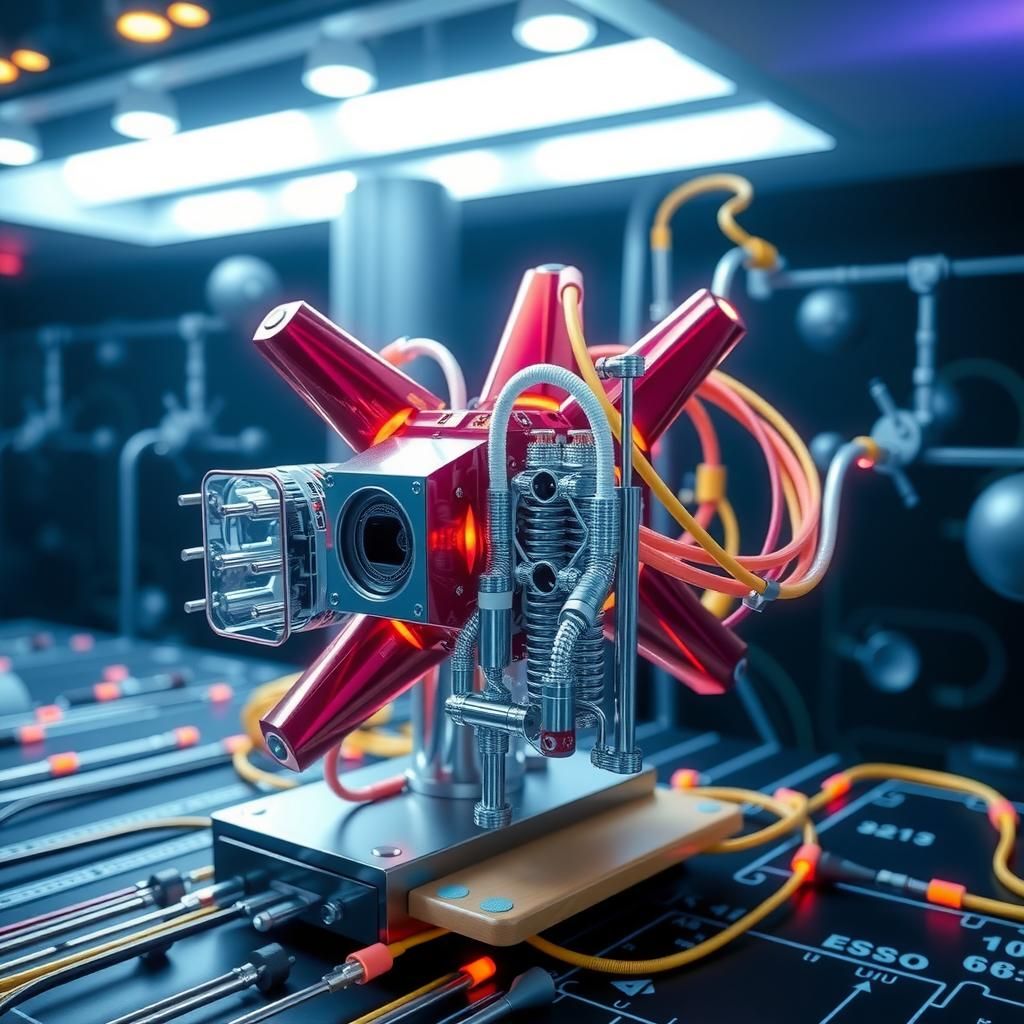
Breakthrough Technologies
Emerging technologies are promising more sustainable AI development:
- Quantum Computing: Potentially reducing energy consumption dramatically
- Edge Computing: Distributing computational load more efficiently
- Neuromorphic Computing: Mimicking brain-like energy efficiency
Research and Academic Initiatives
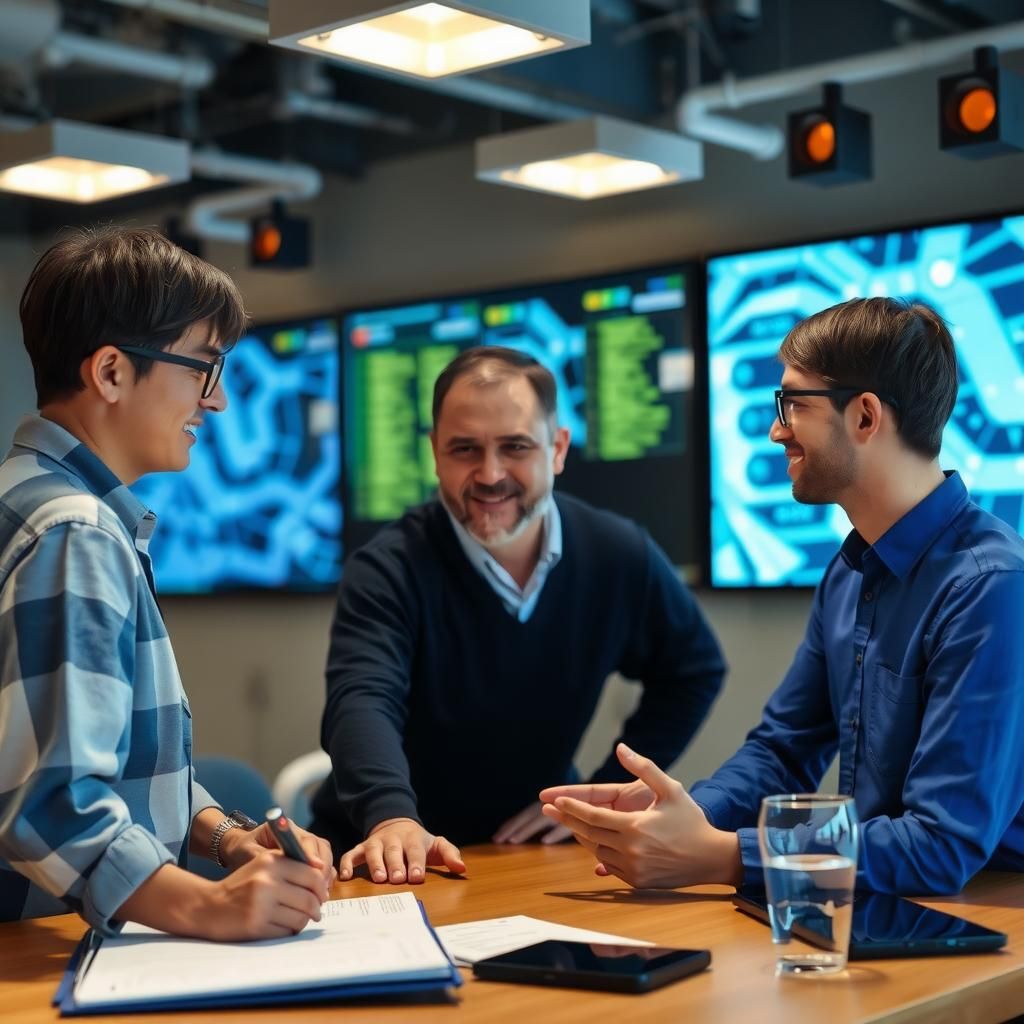
Academic institutions like Stanford's Human-Centered AI Institute are pioneering research into sustainable AI development, focusing on:
- Reducing computational complexity
- Developing more energy-efficient algorithms
- Creating comprehensive environmental impact assessment frameworks
Corporate Responsibility and Transparency
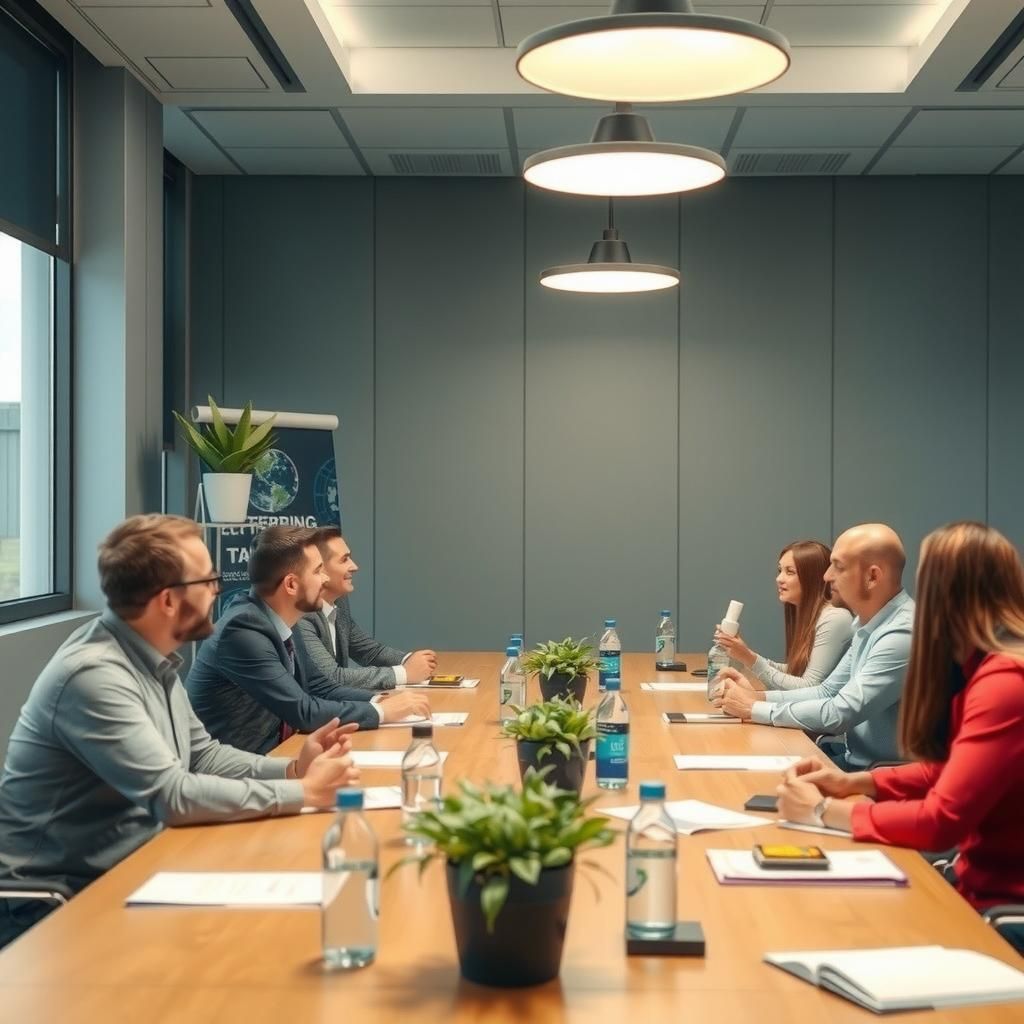
"The future of AI isn't just about technological capability, but about responsible and sustainable innovation." - Leading AI Ethics Researcher
Major tech companies are increasingly recognizing their environmental responsibility, implementing:
- Comprehensive carbon reporting
- Transparent energy consumption metrics
- Aggressive renewable energy targets
Policy and Regulatory Landscape
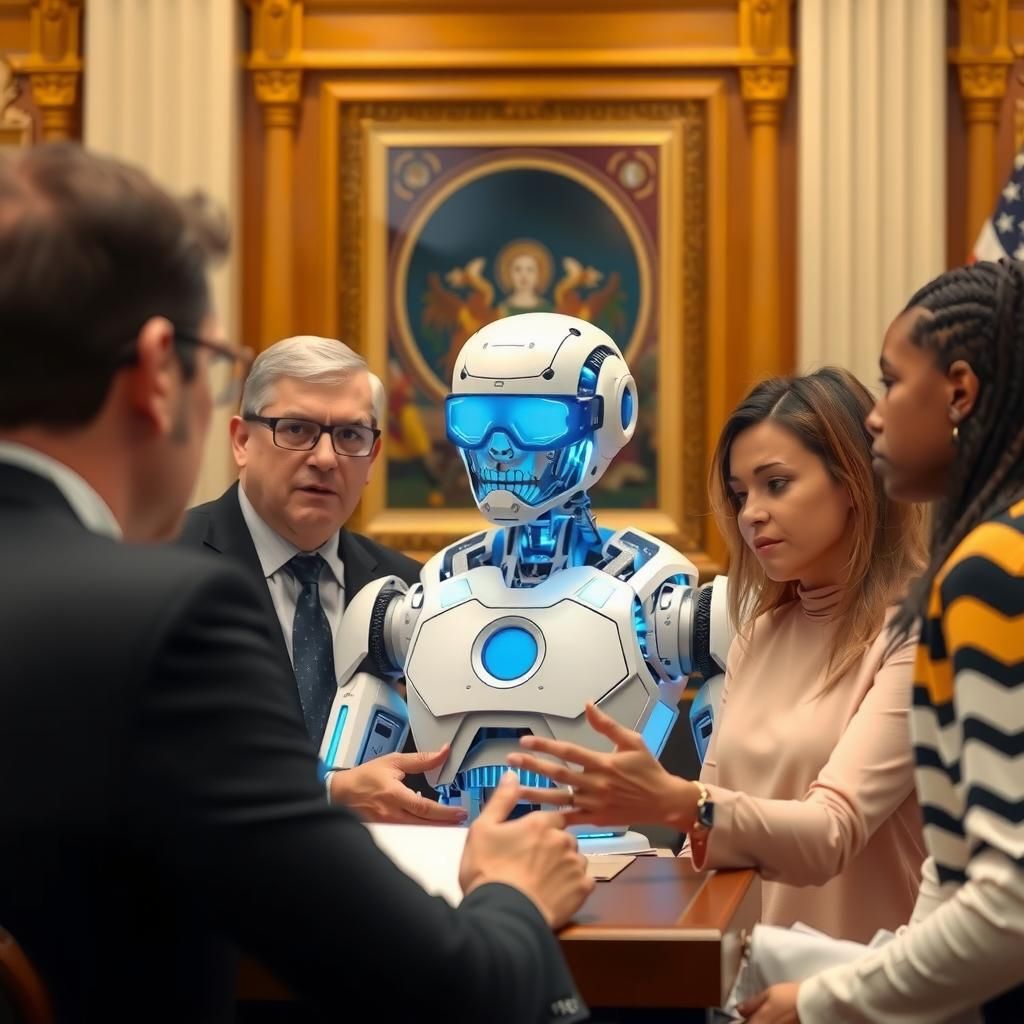
Governments and international bodies are beginning to develop frameworks to address AI's environmental impact:
- Carbon taxation for computational resources
- Mandatory environmental impact assessments
- Incentives for green computing technologies
Future Outlook: Sustainable AI Development
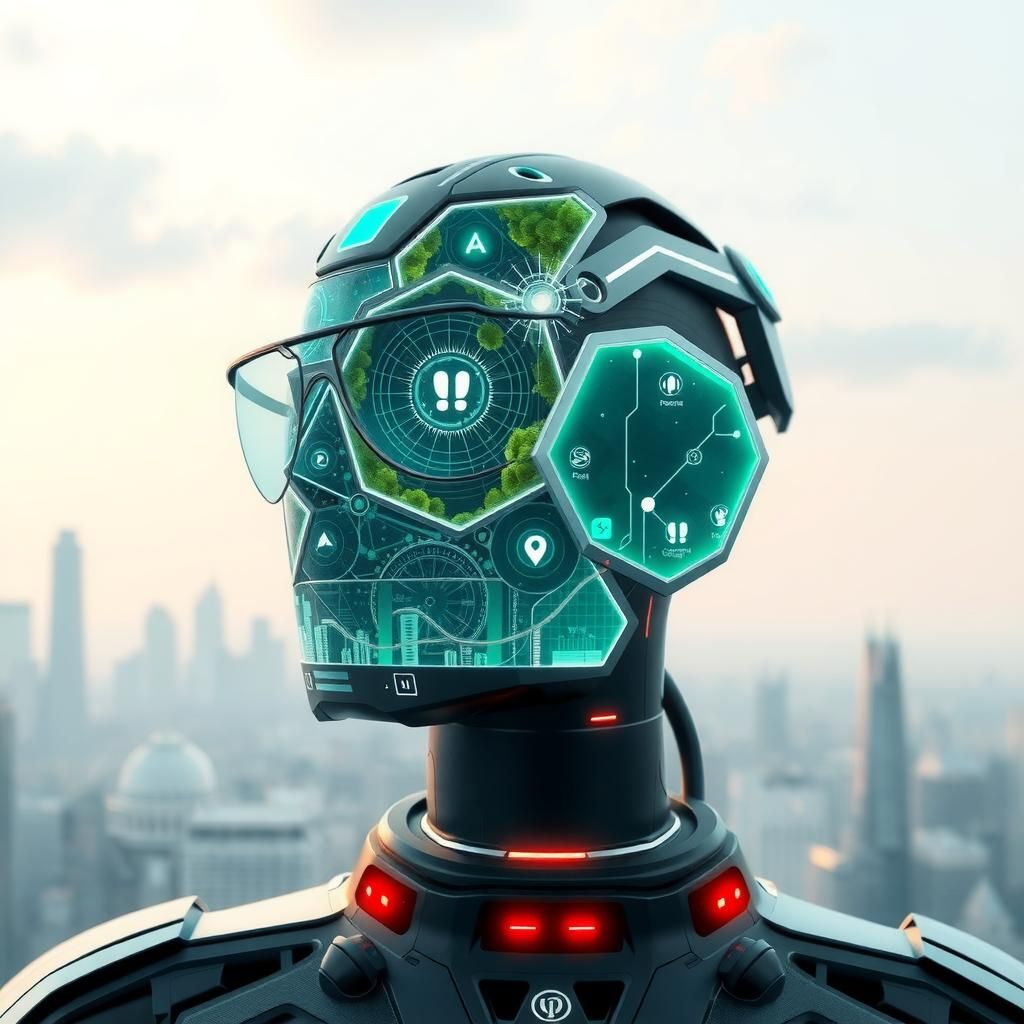
Predictions and Potential
The next decade of AI development will likely focus on:
- Radical energy efficiency improvements
- Decentralized and distributed computing models
- Circular economy principles in AI infrastructure
Frequently Asked Questions
Q1: How Significant is the Environmental Impact of LLMs?
The environmental impact is substantial, with a single model's training potentially generating hundreds of tons of CO2 emissions.
Q2: Are There Practical Solutions to Reduce This Impact?
Yes, solutions include renewable energy, more efficient algorithms, advanced hardware, and carbon offset programs.