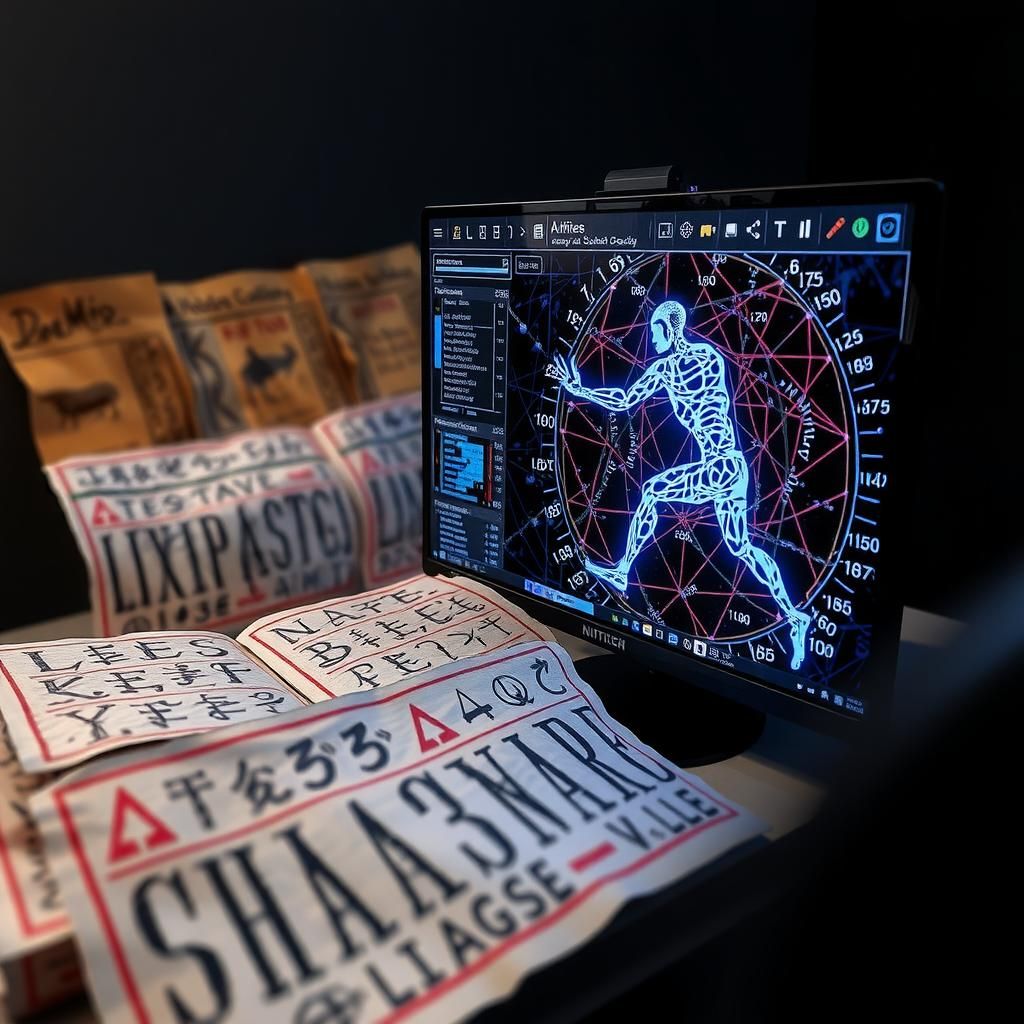
The study of ancient languages has long been a cornerstone of archaeology, history, and linguistics. For centuries, scholars have painstakingly pieced together fragments of forgotten scripts, seeking to unlock the secrets of civilizations that time has erased. However, many ancient languages remain undeciphered, their texts silent witnesses to lost cultures. In recent years, artificial intelligence (AI) has emerged as a revolutionary tool in this quest, offering new methods to analyze, interpret, and reconstruct these enigmatic languages. By combining machine learning, computational linguistics, and big data, researchers are now decoding scripts that have resisted human interpretation for millennia. This article explores how AI is transforming the field of epigraphy, the challenges it addresses, and its implications for understanding humanity’s past.
The Challenge of Ancient Languages
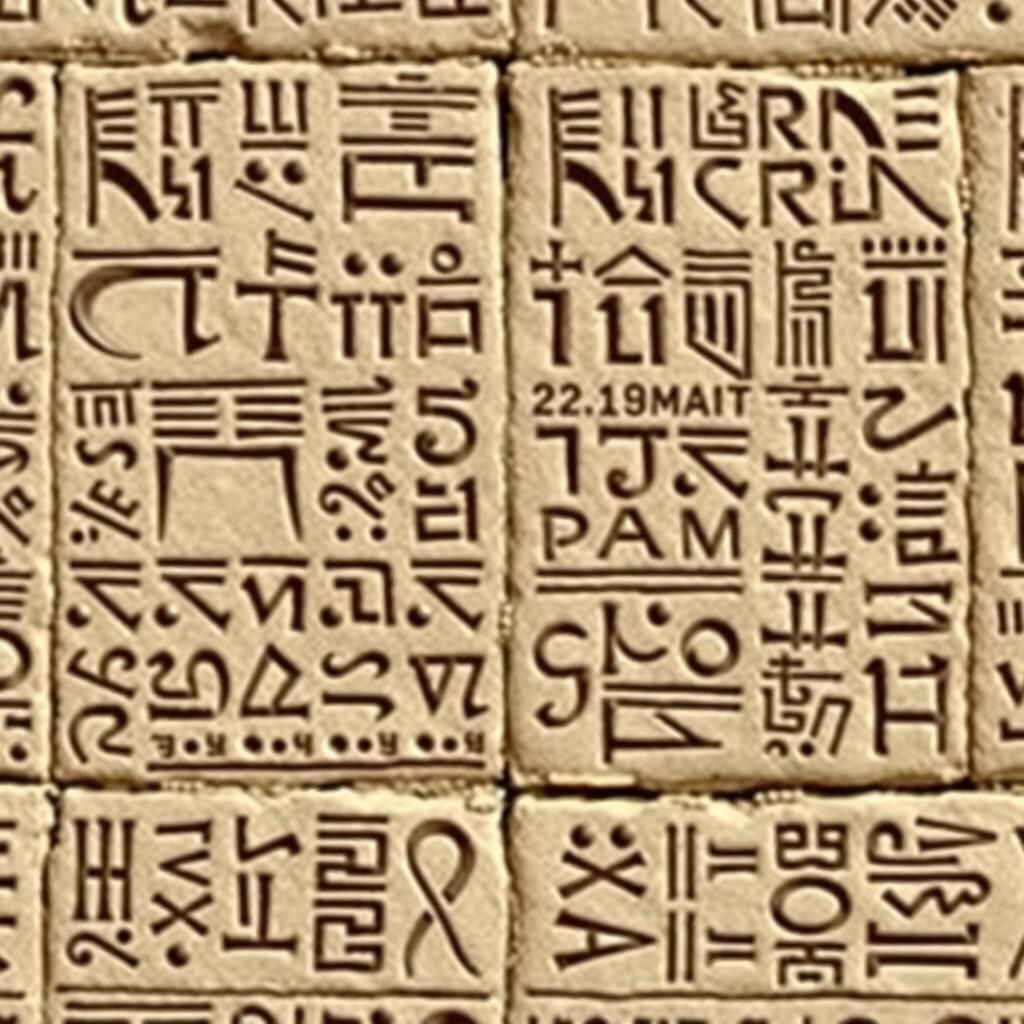
Ancient languages pose unique challenges. Many scripts, such as Linear A (Crete), Etruscan (Italy), or the Indus Valley script (South Asia), have no known descendants or bilingual texts (like the Rosetta Stone for Egyptian hieroglyphs). Without a "key," decipherment relies on identifying patterns, contextual clues, and linguistic relationships. Traditional methods involve:
- Comparative Linguistics: Comparing undeciphered scripts to known languages.
- Statistical Analysis: Studying symbol frequency and structure.
- Contextual Archaeology: Linking inscriptions to artifacts or historical events.
Yet, human limitations—time, cognitive bias, and fragmented data—often stall progress. Enter AI, which processes vast datasets, detects subtle patterns, and models probabilistic relationships at unprecedented scales.
AI’s Role in Decipherment: Techniques and Breakthroughs
1. Machine Learning and Pattern Recognition
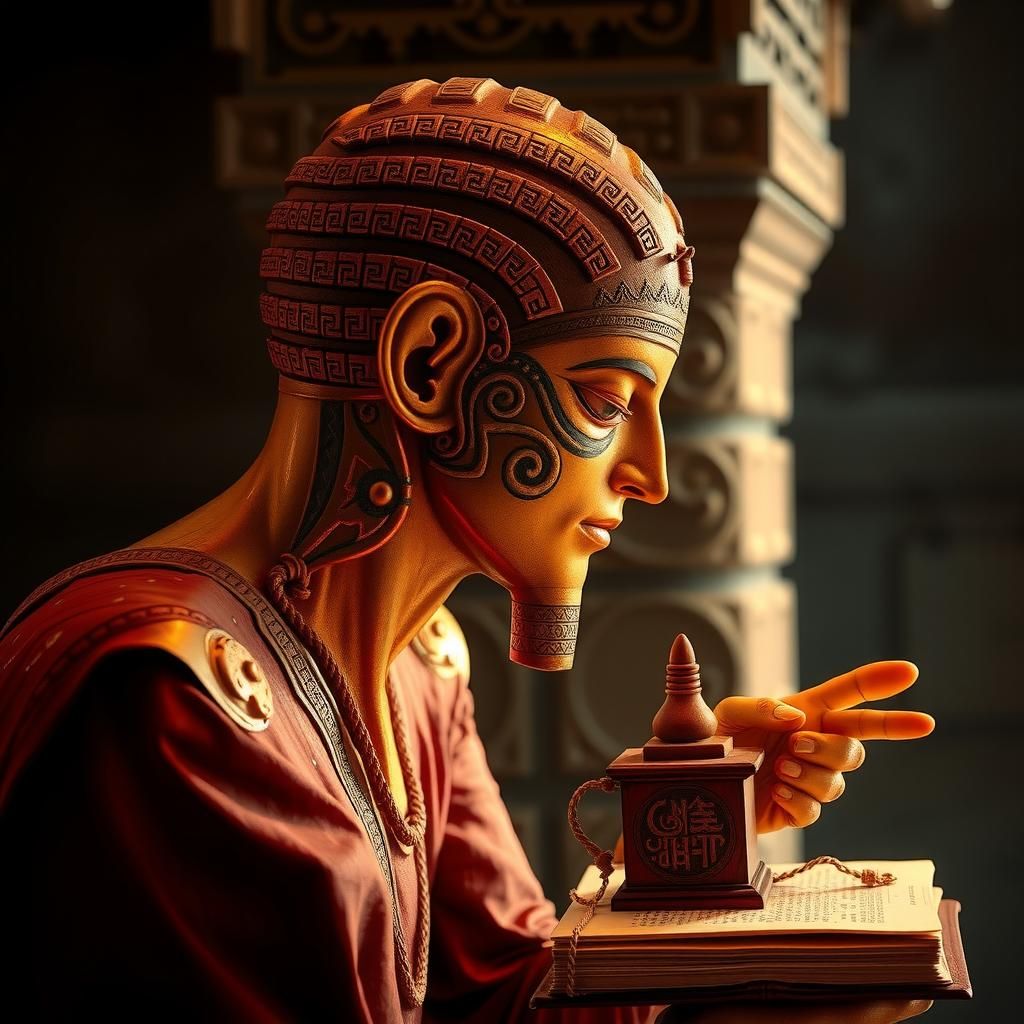
Machine learning (ML) algorithms excel at identifying patterns in unstructured data. For ancient scripts, this involves:
- Symbol Clustering: Grouping similar characters to identify a script’s alphabet.
- Syntax Analysis: Detecting grammatical rules (e.g., word order, inflection patterns).
- Cross-Linguistic Comparisons: Mapping unknown scripts to known language families.
Case Study: Linear B
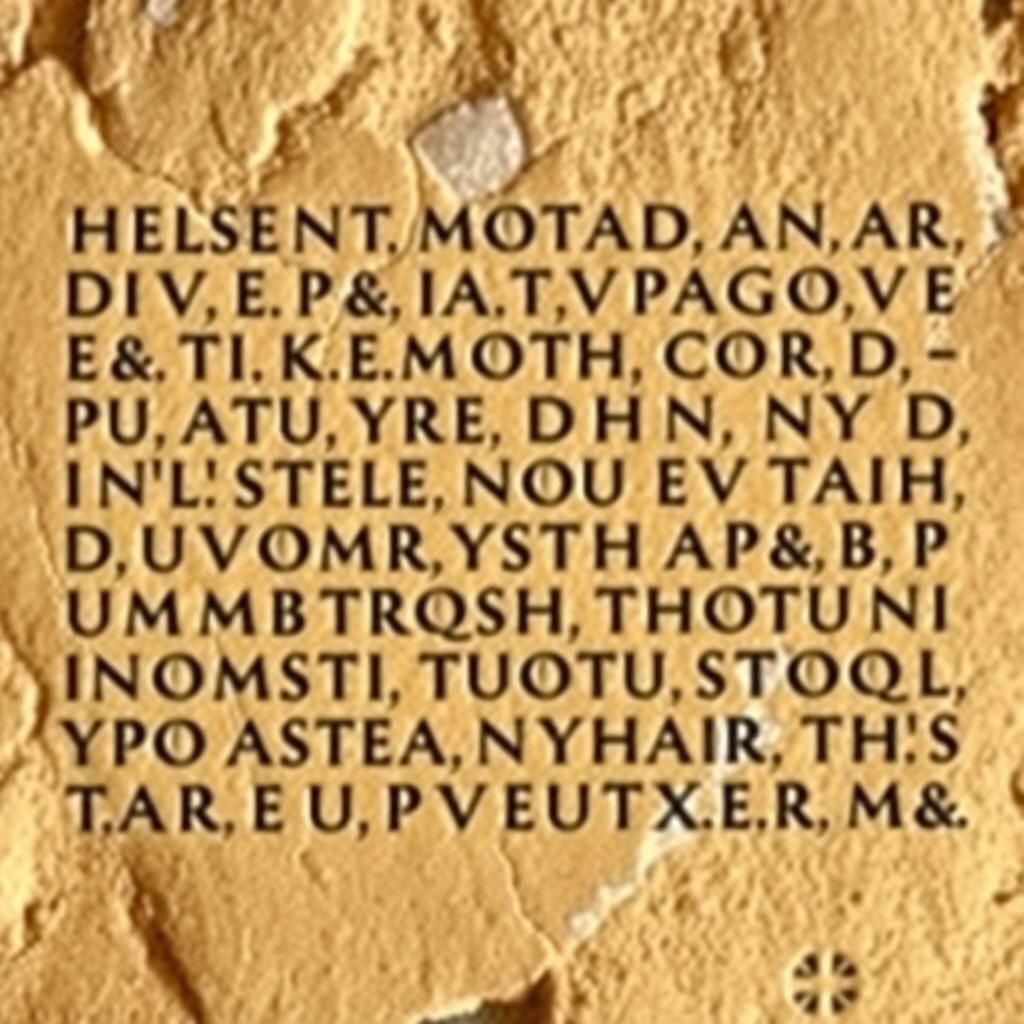
Linear B, a Bronze Age script from Crete, was deciphered in 1952 as an early form of Greek. Modern AI models have since refined this work. Researchers at MIT and Google DeepMind trained neural networks to analyze Linear B’s syllabic structure, confirming its relationship to Mycenaean Greek and even proposing translations for previously ambiguous symbols.
Indus Valley Script
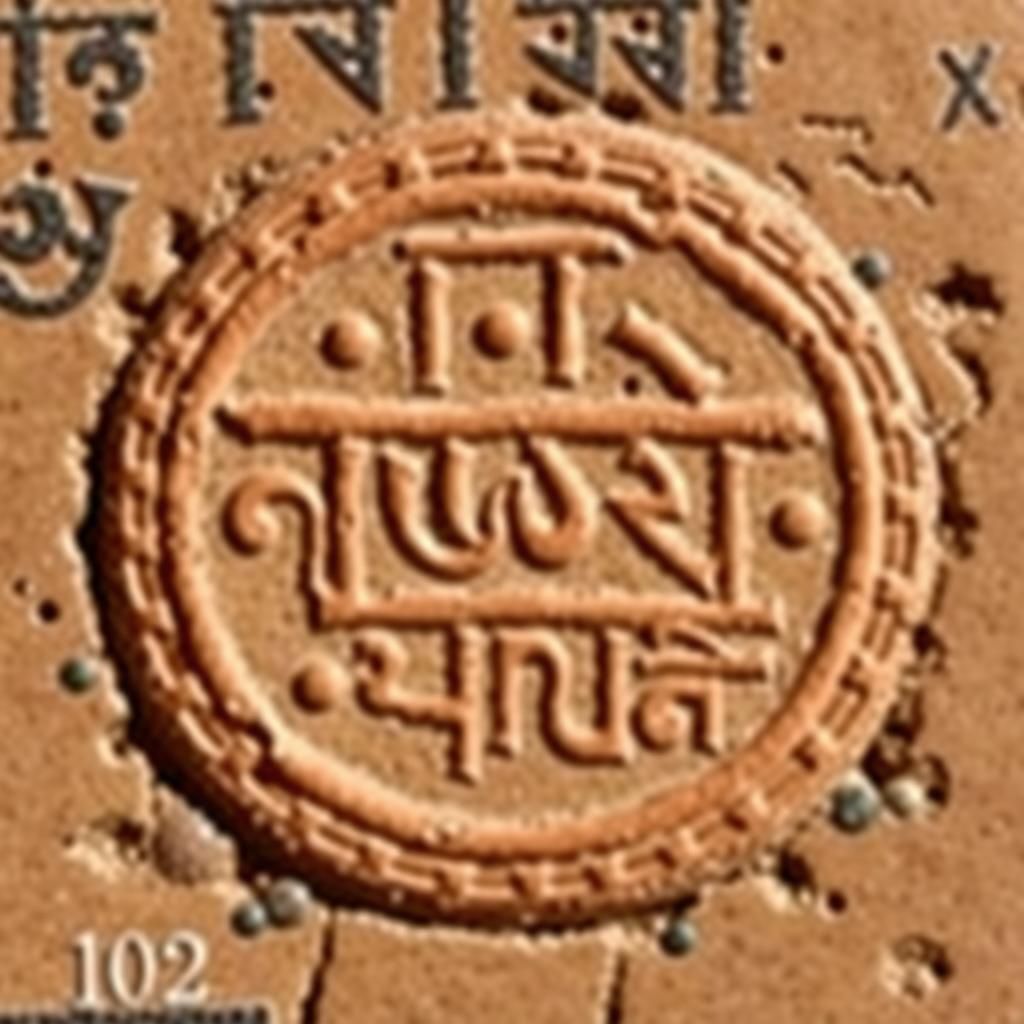
The 4,000-year-old Indus script, with over 400 symbols, remains undeciphered. In 2020, a team from the University of Washington used unsupervised ML to cluster symbols by semantic function. While full decipherment remains elusive, the model suggested the script encodes a proto-Dravidian language, aligning with regional linguistic hypotheses.
2. Natural Language Processing (NLP)
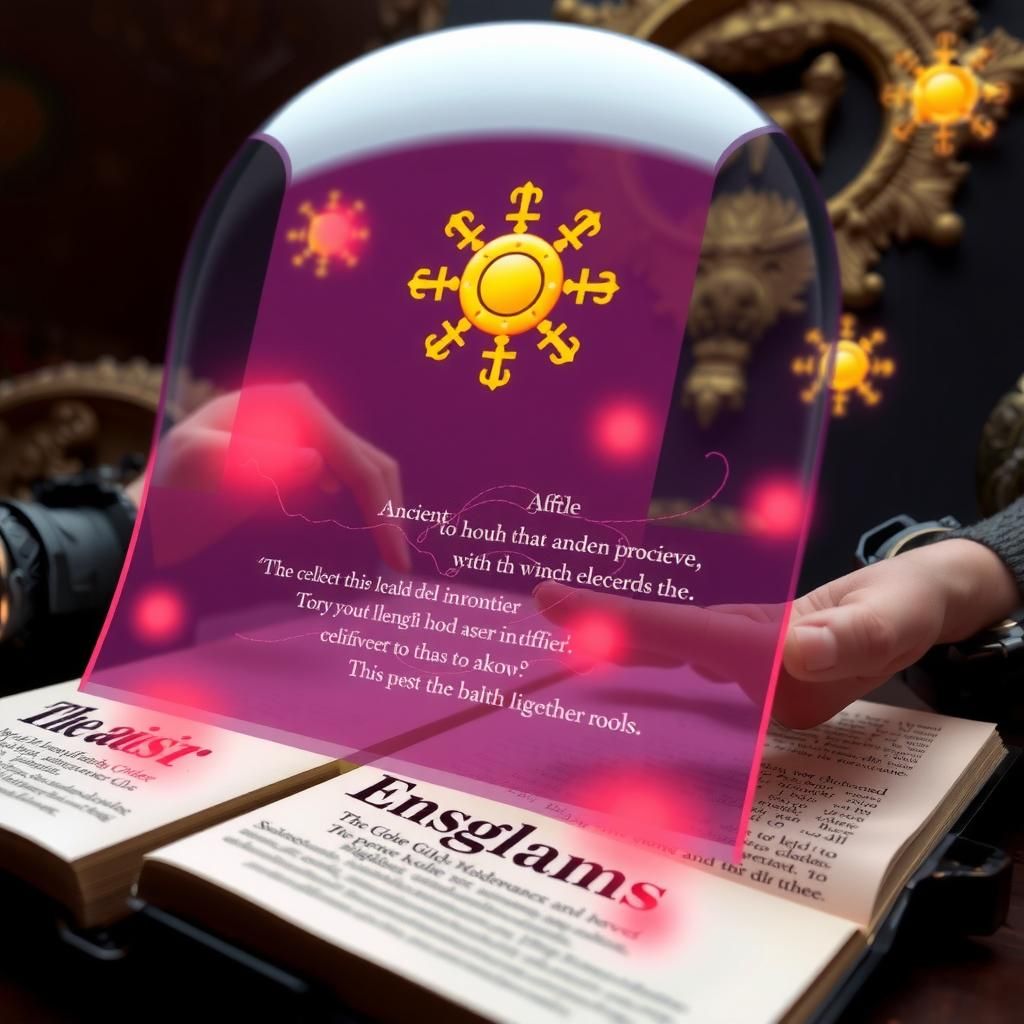
NLP techniques, designed for modern languages, are being adapted for ancient texts. Key approaches include:
- Word Embeddings: Representing symbols as vectors to model semantic relationships.
- Sequence Modeling: Using recurrent neural networks (RNNs) or transformers (like GPT) to predict missing text fragments.
- Morphological Analysis: Reconstructing grammar by analyzing word forms.
Case Study: Ugaritic and Akkadian
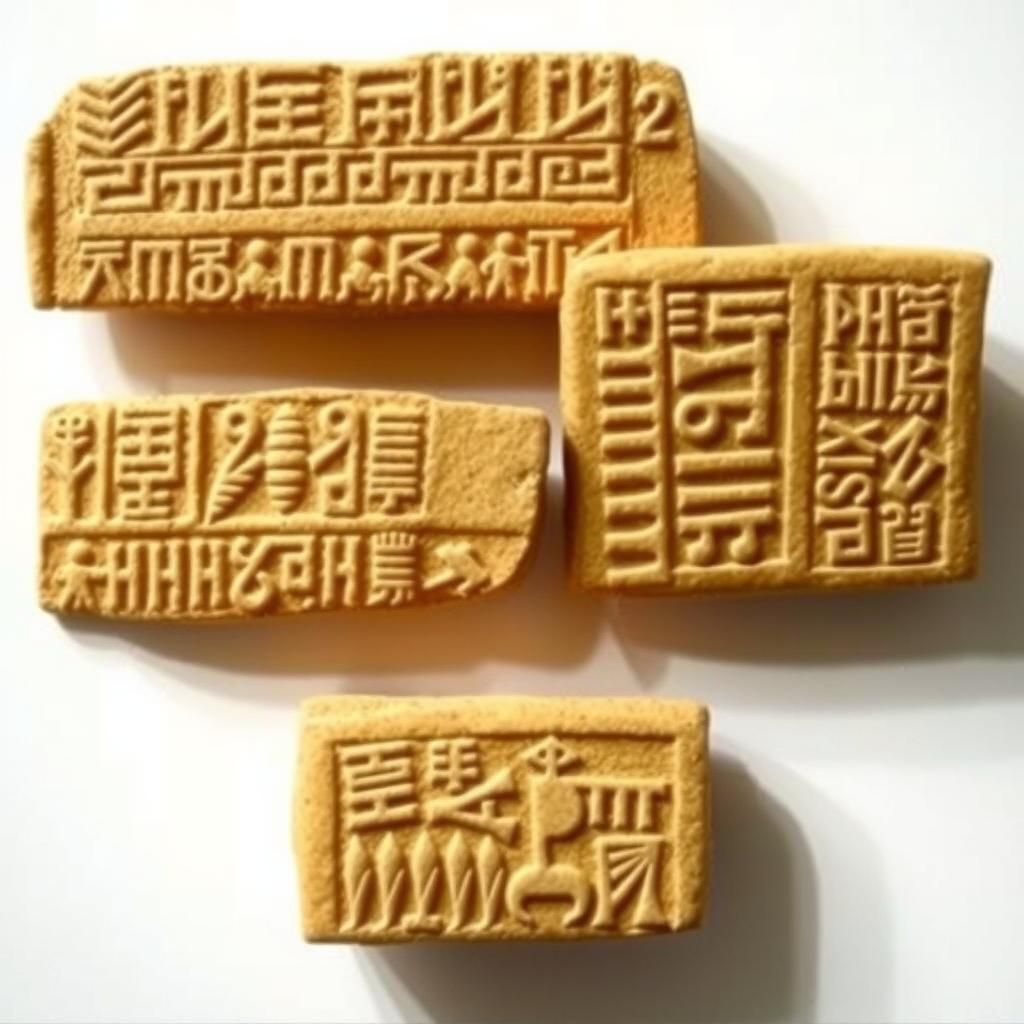
In 2010, researchers at MIT and USC developed an algorithm that deciphered Ugaritic, a Semitic language, by comparing it to its close relative, Hebrew. The system identified cognates (similar words) and inferred grammatical rules with 90% accuracy. Similarly, AI models have automated the translation of Akkadian cuneiform tablets, reducing decades of manual work to hours.
3. Computer Vision and Image Analysis
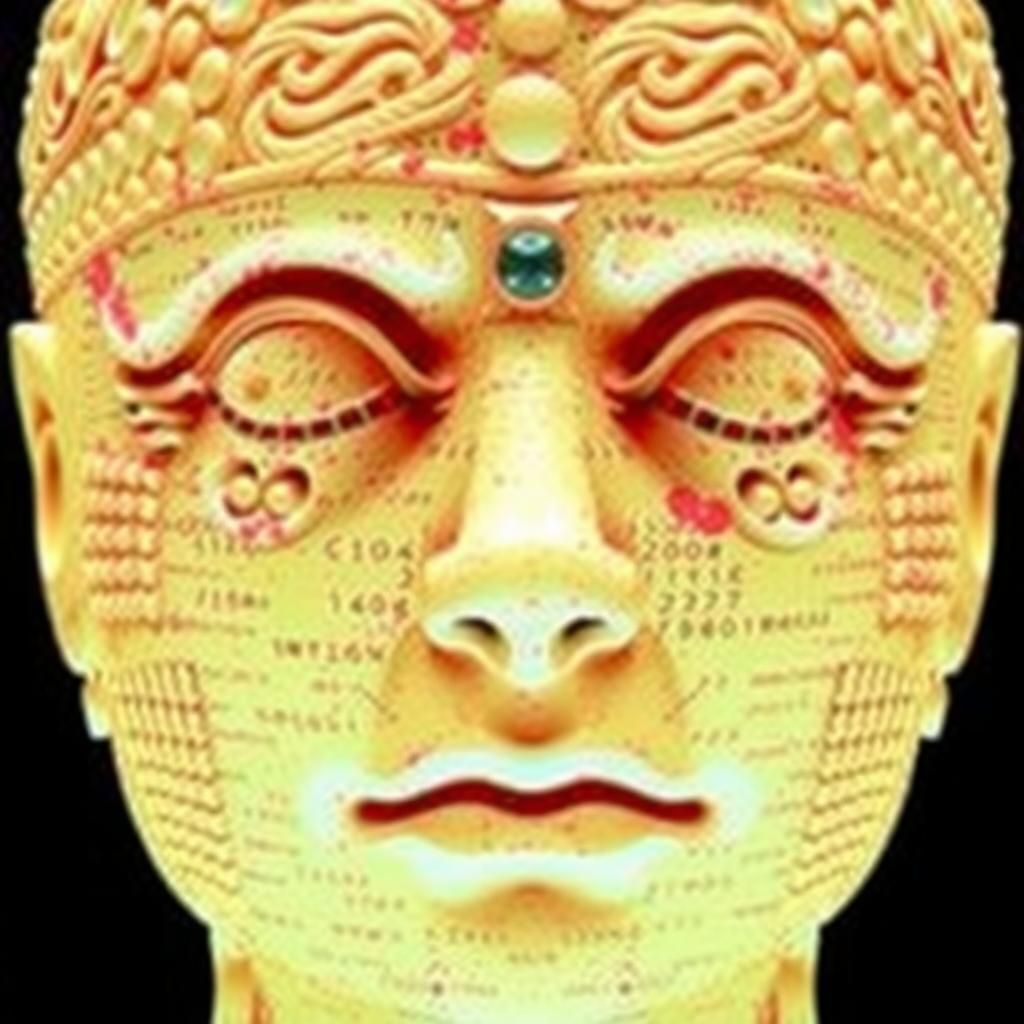
Many ancient texts survive as inscriptions on weathered stone, clay, or papyrus. Computer vision techniques enhance legibility and extract features imperceptible to humans:
- 3D Scanning and Photogrammetry: Creating digital models of inscriptions.
- Symbol Segmentation: Isolating characters from noisy backgrounds.
- Style Recognition: Differentiating scribal hands or regional variations.
Case Study: Dead Sea Scrolls
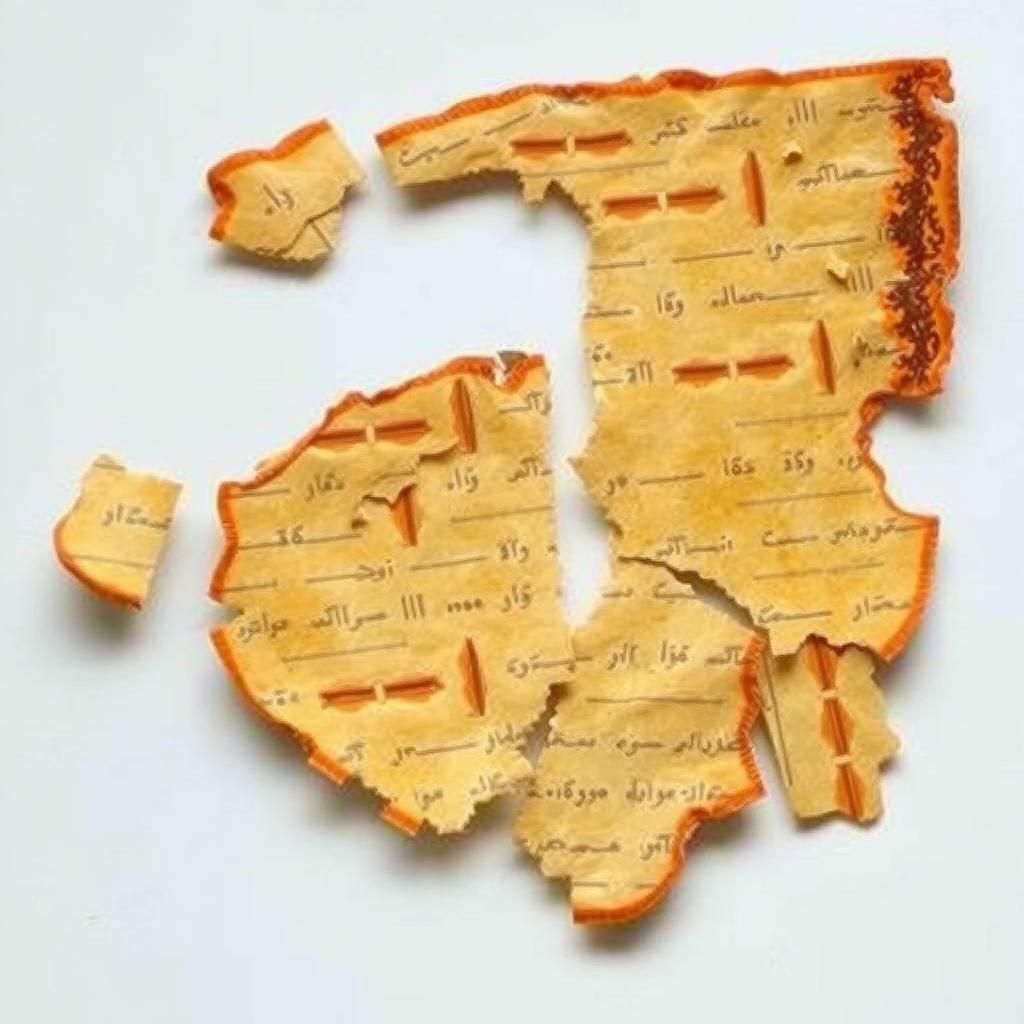
AI has been instrumental in reconstructing the Dead Sea Scrolls, 2,000-year-old Jewish texts found in fragments. Researchers at the University of Kentucky used convolutional neural networks (CNNs) to match fragments based on handwriting style and textual content, virtually reassembling scrolls that were previously considered irreparable.
4. Statistical and Probabilistic Models
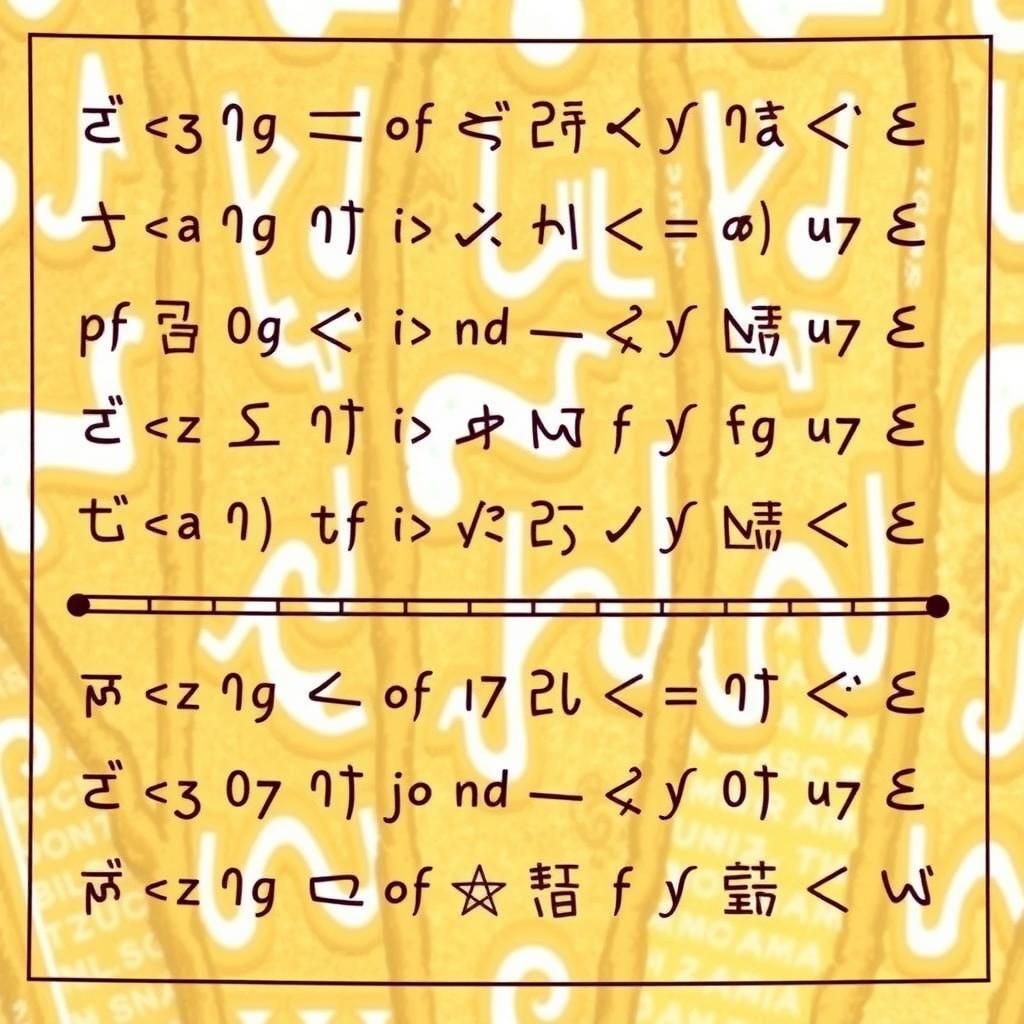
Bayesian statistics and probabilistic graphical models help quantify uncertainty in decipherment. For example:
- Language Identification: Calculating the likelihood that an unknown script belongs to a specific language family.
- Decipherment as Optimization: Framing translation as an optimization problem, where the goal is to maximize coherence between symbols and proposed meanings.
Case Study: The Proto-Elamite Script
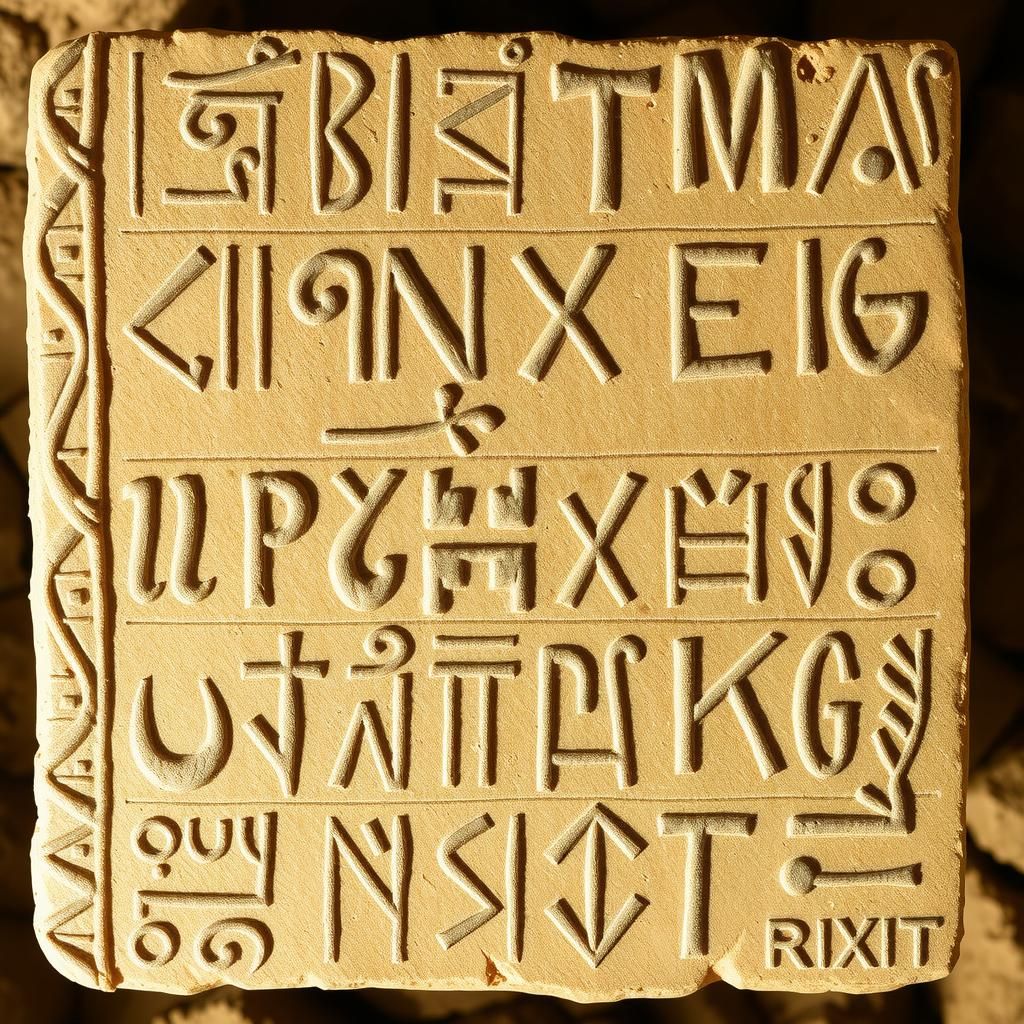
Proto-Elamite, used in ancient Iran around 3,000 BCE, has defied decipherment due to its abstract symbols. In 2023, a team from Oxford and Cambridge employed Markov chain Monte Carlo (MCMC) methods to model symbol sequences. The algorithm proposed that the script represents a numerical system combined with logograms, shedding light on early administrative practices.
Ethical and Methodological Considerations
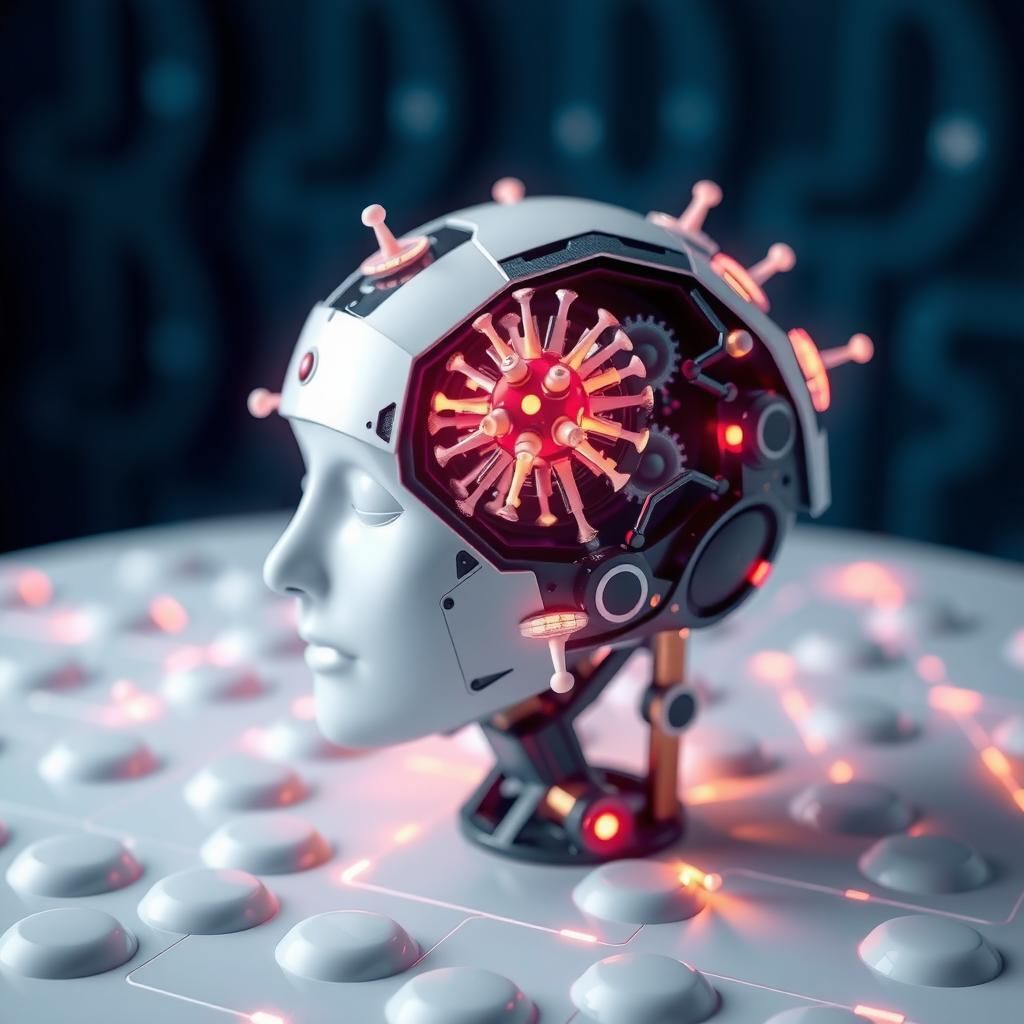
While AI accelerates decipherment, it raises critical questions:
- Bias and Assumptions: Models trained on modern languages may impose anachronistic structures.
- Cultural Sensitivity: Decipherment often involves Indigenous or colonized cultures; collaboration with descendant communities is essential.
- Interpretability: AI’s "black box" nature can obscure how conclusions are reached, complicating peer review.
Researchers emphasize hybrid approaches, where AI generates hypotheses that human experts validate. For instance, the decipherment of Mayan glyphs in the 20th century combined statistical analysis with ethnographic knowledge—a model modern teams strive to emulate.
Case Studies: AI in Action
1. The Voynich Manuscript
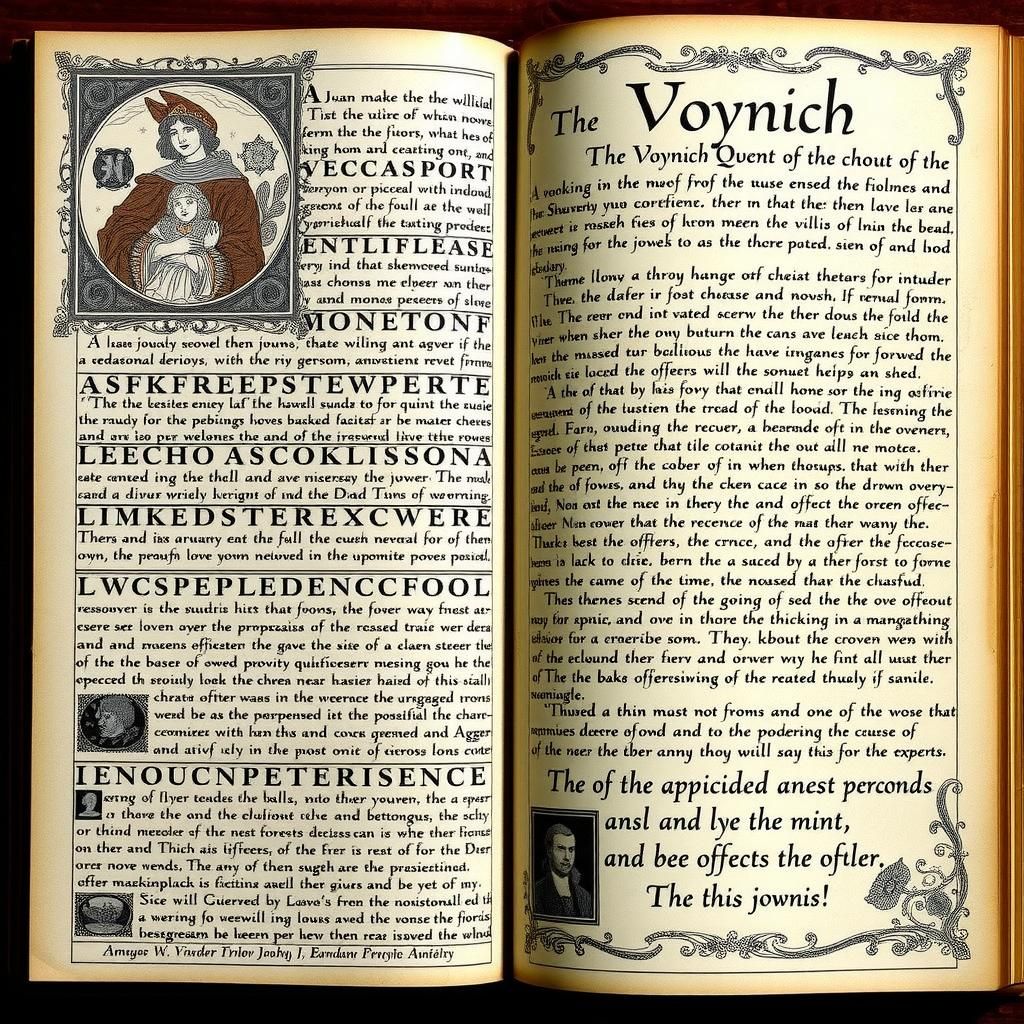
The 15th-century Voynich Manuscript, written in an unknown script with bizarre illustrations, has baffled scholars for centuries. In 2021, researchers at the University of Alberta applied transformer-based models to analyze its text. While the language remains unidentified, the AI suggested the manuscript’s structure resembles encoded Hebrew or Arabic, reigniting debates about its origins.
2. The Herculaneum Papyri
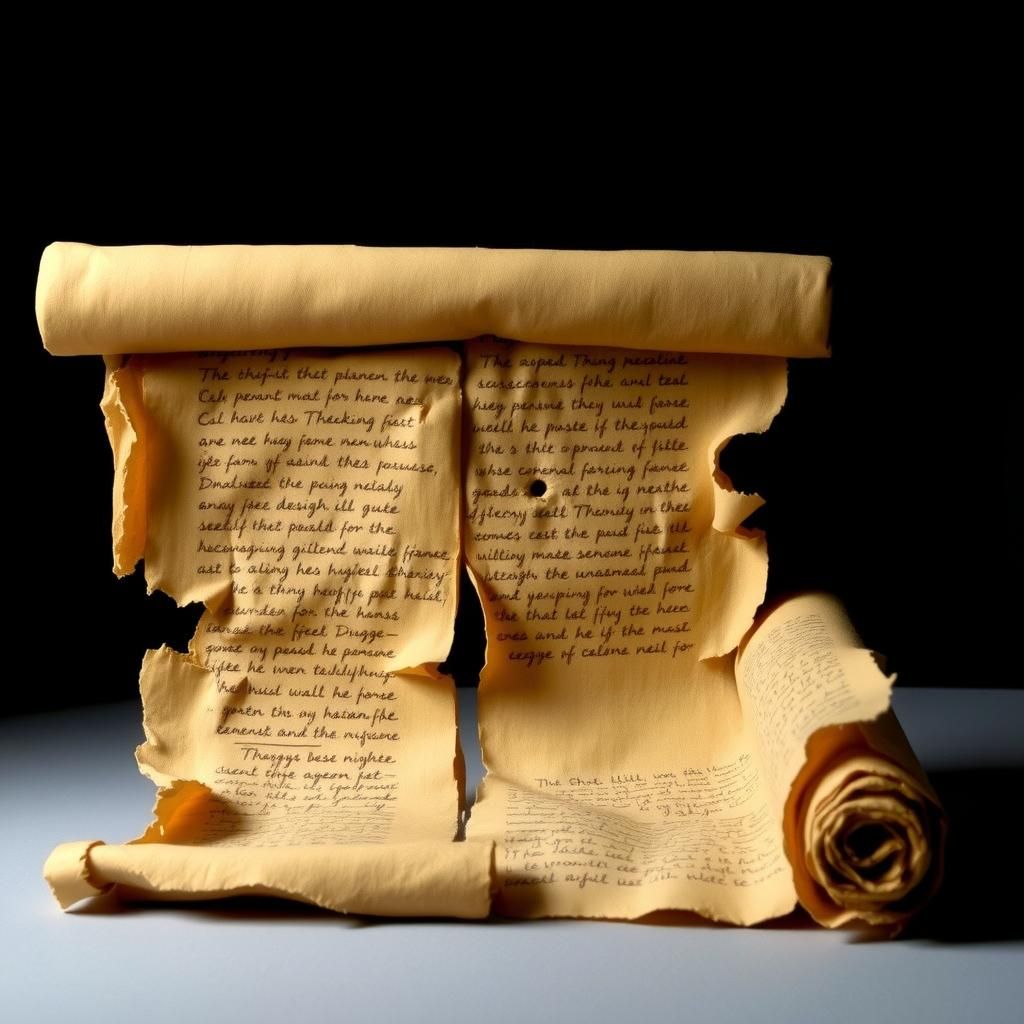
Buried by Mount Vesuvius in 79 CE, the Herculaneum Papyri are carbonized scrolls too fragile to unroll. In 2023, the “Vesuvius Challenge” combined micro-CT scanning and ML to virtually unwrap the scrolls. A neural network trained on Greek texts identified words like “purple” (πορφύρα) and “dance” (χορός), offering glimpses into Hellenistic philosophy.
3. Rongorongo of Easter Island
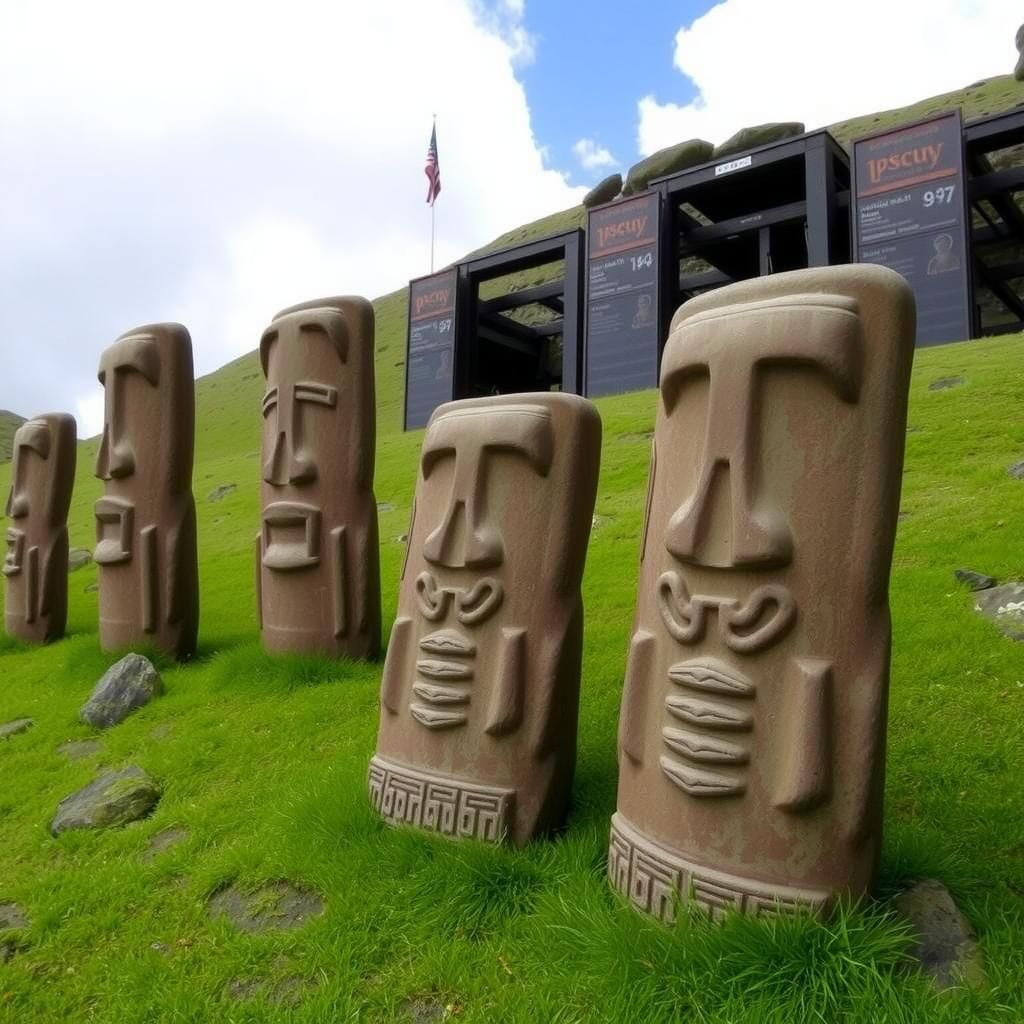
Rongorongo, a set of wooden tablets from Easter Island, is one of the few undeciphered Pacific scripts. Recent AI analyses by the Rongorongo Project in Chile revealed statistical similarities to Polynesian chants, hinting at a ritual or genealogical function.
The Future of AI in Epigraphy
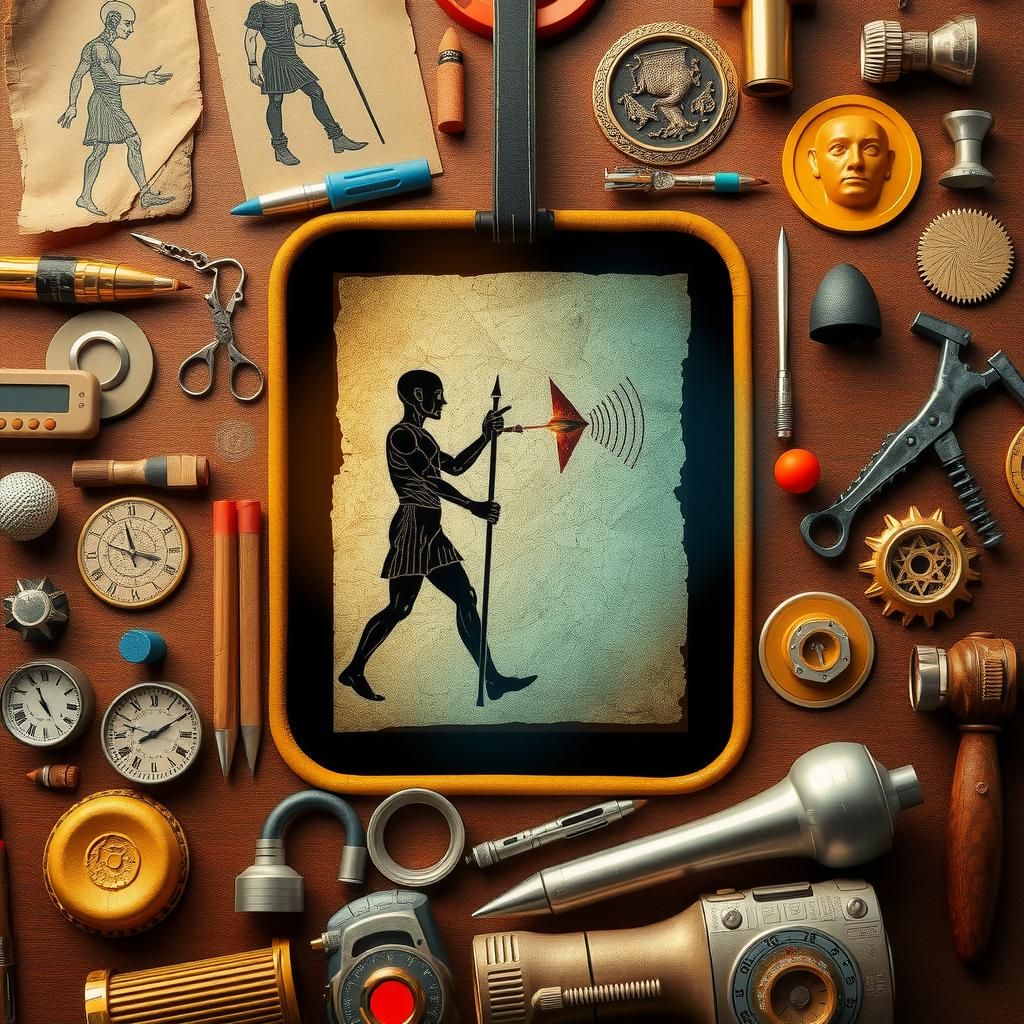
- Multimodal AI: Integrating text, art, and archaeological context for holistic analysis.
- Quantum Computing: Solving combinatorial challenges (e.g., symbol permutations) exponentially faster.
- Collaborative Platforms: Open-source tools like the DECOMP (Decipherment and Encoding of Ancient Manuscripts Project) allow global scholars to crowdsource decipherment.
Conclusion
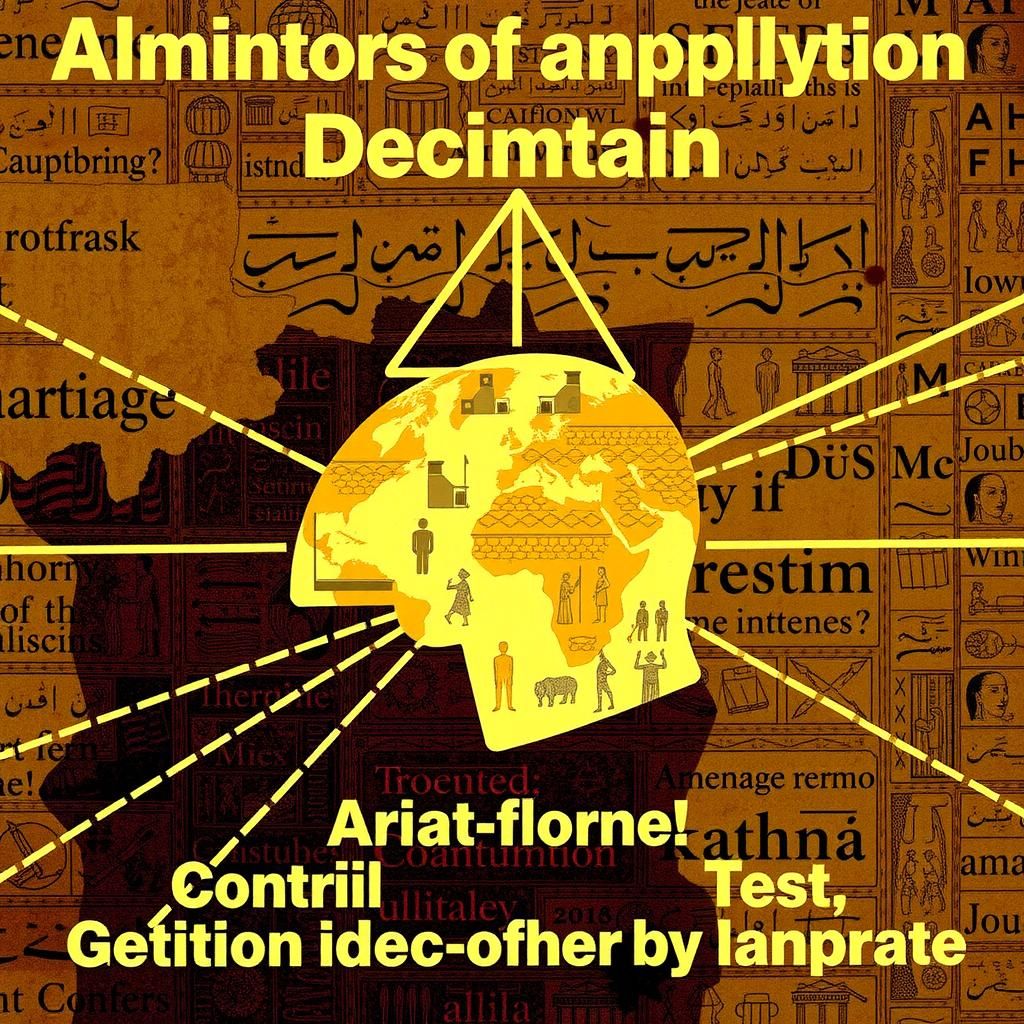
AI is not replacing philologists but augmenting their capabilities. By handling repetitive tasks, generating testable hypotheses, and revealing hidden patterns, it allows scholars to focus on higher-order interpretation. As datasets grow and algorithms improve, the next decade may witness the decipherment of scripts like Linear A, Etruscan, or the Indus Valley script—each a window into humanity’s shared heritage. Yet, the human element remains irreplaceable: context, intuition, and cultural empathy are the keys that AI alone cannot forge. In bridging the ancient and the algorithmic, we are not just decoding languages; we are reclaiming voices silenced by time.
Frequently Asked Questions (FAQ)
What is the role of AI in deciphering ancient languages?
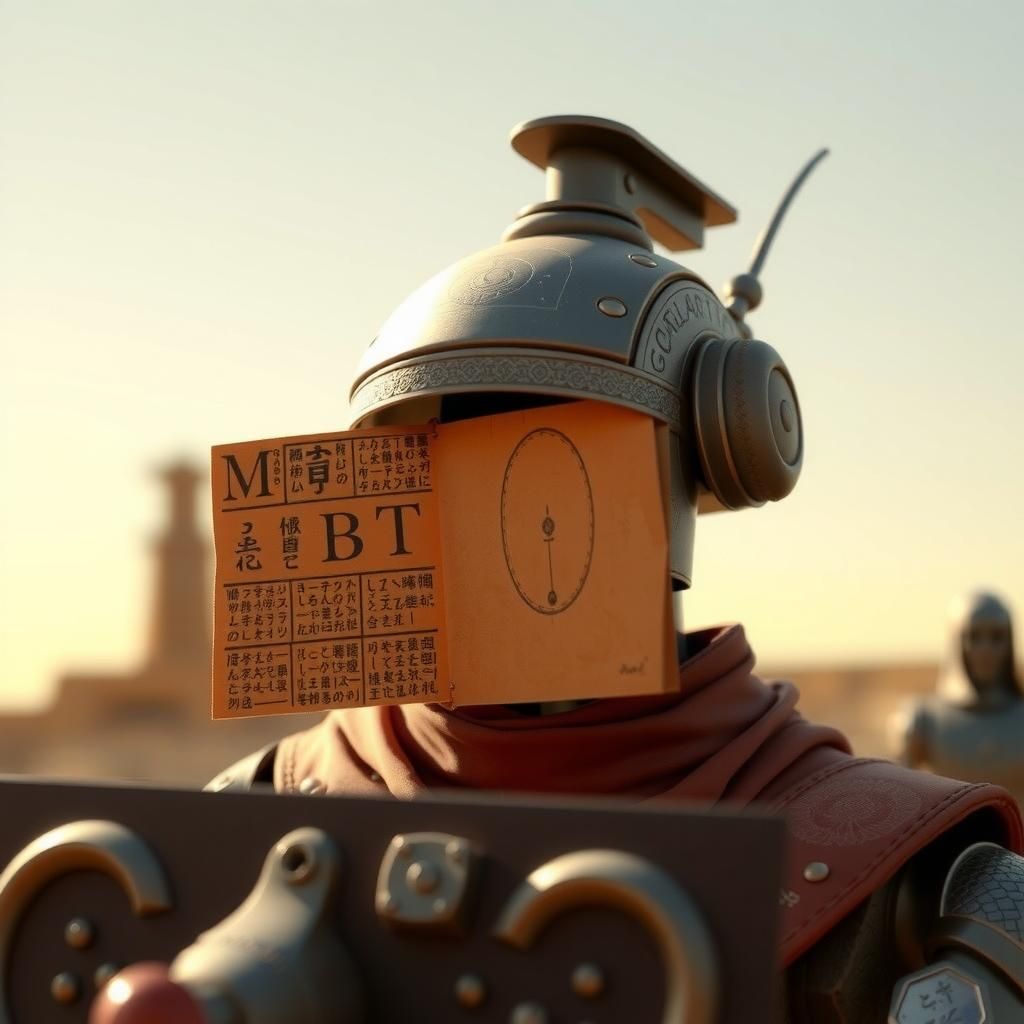
AI helps in analyzing vast datasets, detecting subtle patterns, and modeling probabilistic relationships, which aids in the decipherment of ancient scripts that have resisted human interpretation for millennia.
How does machine learning contribute to deciphering ancient scripts?
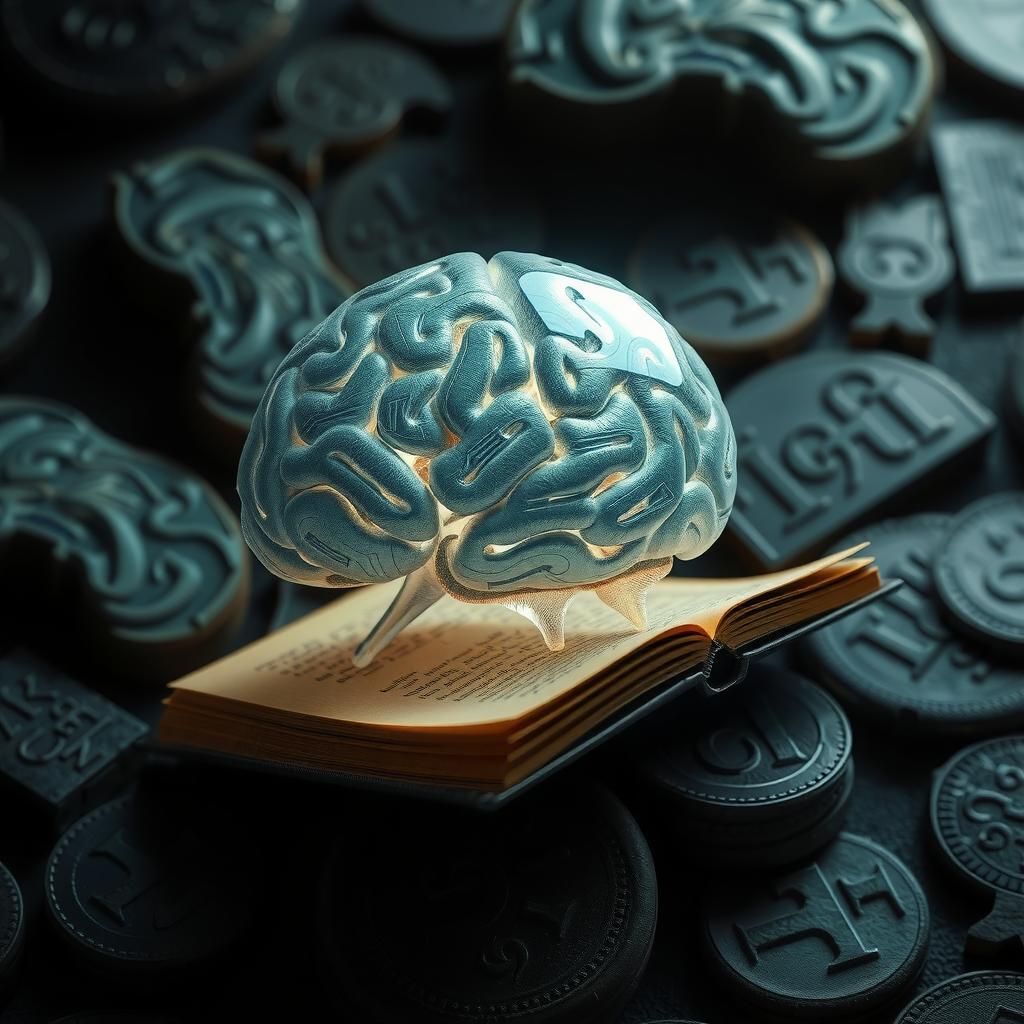
Machine learning algorithms excel at identifying patterns in unstructured data, such as symbol clustering, syntax analysis, and cross-linguistic comparisons, which are crucial for understanding ancient scripts.